Exploring QSAR of FLAP inhibitors using kernel partial least squares modeling: Insights from molecular binary fingerprints
Abstract
FLAP (5-Lipoxygenase Activating Protein) inhibitors, offering targeted intervention in leukotriene biosynthesis and holding therapeutic promise for inflammatory diseases like asthma, are hindered by current inhibitors' off-target effects, limited efficacy, safety concerns, potential drug interactions, and accessibility issues. Given these challenges, computational methods, particularly Quantitative Structure Activity Relationships (QSAR) modeling, are vital for developing novel FLAP inhibitors. This study specifically investigates the QSAR of FLAP inhibitors using Kernel Partial Least Squares (KPLS) modeling. Leveraging a dataset of FLAP inhibitors, we employ KPLS within the Schrödinger Canvas environment to correlate molecular descriptors with biological activity. Out of the eight models developed, the "atom pairs" fingerprint yielded a statistically significant 2D QSAR model with outstanding regression coefficient values (R2=0.9624). The model demonstrated high predictive ability for external test set data (R2pred = 0.7105), underscoring its robustness and reliability in accurately predicting biological activity based on molecular structure. Additionally, we tried to visualize the relative contributions of individual atoms within FLAP inhibitors, providing insights into their favorable and unfavorable characteristics. Through the analysis of atomic contributions, we identify key structural motifs crucial for predicting FLAP inhibitor activity. Our findings not only advance our understanding of FLAP inhibitor SAR but also demonstrate the utility of KPLS modeling and atomic contribution analysis in drug discovery efforts. Furthermore, this study contributes to the development of anti-inflammatory therapeutics by elucidating the structural determinants of FLAP inhibitor activity, with potential applications in the treatment of inflammatory disorders.
References
K. A. Heidenreich and C. E. Corser-Jensen, “Chapter 12 - 5-Lipoxygenase-Activating Protein Inhibitors: Promising Drugs for Treating Acute and Chronic Neuroinflammation Following Brain Injury,” in New Therapeutics for Traumatic Brain Injury, K. A. Heidenreich, Ed., San Diego: Academic Press, 2017, pp. 199–210. https://doi.org/https://doi.org/10.1016/B978-0-12-802686-1.00012-2
D. Pettersen et al., “Discovery and Early Clinical Development of an Inhibitor of 5-Lipoxygenase Activating Protein (AZD5718) for Treatment of Coronary Artery Disease,” J Med Chem, vol. 62, no. 9, pp. 4312–4324, May 2019, https://doi.org/10.1021/acs.jmedchem.8b02004
Z. T. Gür, B. Çalışkan, and E. Banoglu, “Drug discovery approaches targeting 5-lipoxygenase-activating protein (FLAP) for inhibition of cellular leukotriene biosynthesis,” Eur J Med Chem, vol. 153, pp. 34–48, 2018, https://doi.org/https://doi.org/10.1016/j.ejmech.2017.07.019
A. Cherkasov et al., “QSAR Modeling: Where have you been? Where are you going to?,” vol. 57, no. 12, pp. 4977–5010, 2015, https://doi.org/10.1021/jm4004285.QSAR
A. Golbraikh, X. S. Wang, H. Zhu, and A. Tropsha, “Predictive QSAR Modeling: Methods and Applications in Drug Discovery and Chemical Risk Assessment BT - Handbook of Computational Chemistry,” J. Leszczynski, Ed., Springer Netherlands, 2016, pp. 1–38. https://doi.org/10.1007/978-94-007-6169-8_37-2
E. N. Muratov et al., “QSAR without borders,” Chem Soc Rev, vol. 49, no. 11, pp. 3525–3564, 2020, https://doi.org/10.1039/D0CS00098A
J. Yang, Y. Cai, K. Zhao, H. Xie, and X. Chen, “Concepts and applications of chemical fingerprint for hit and lead screening,” Drug Discov Today, vol. 27, no. 11, p. 103356, 2022, https://doi.org/https://doi.org/10.1016/j.drudis.2022.103356
B. Zagidullin, Z. Wang, Y. Guan, E. Pitkänen, and J. Tang, “Comparative analysis of molecular fingerprints in prediction of drug combination effects,” Brief Bioinform, vol. 22, no. 6, p. bbab291, Nov. 2021, https://doi.org/10.1093/bib/bbab291
Á. Orosz, K. Héberger, and A. Rácz, “Comparison of Descriptor- and Fingerprint Sets in Machine Learning Models for ADME-Tox Targets,” Front Chem, vol. 10, 2022, https://doi.org/10.3389/fchem.2022.852893
V. Consonni, D. Ballabio, and R. Todeschini, “Chapter 12 - Chemical space and molecular descriptors for QSAR studies,” in Cheminformatics, QSAR and Machine Learning Applications for Novel Drug Development, K. Roy, Ed., Academic Press, 2023, pp. 303–327. https://doi.org/https://doi.org/10.1016/B978-0-443-18638-7.00022-0
Y. An, W. Sherman, and S. L. Dixon, “Kernel-Based Partial Least Squares: Application to Fingerprint-Based QSAR with Model Visualization,” J Chem Inf Model, vol. 53, no. 9, pp. 2312–2321, Sep. 2013, https://doi.org/10.1021/ci400250c
S. Kim et al., “PubChem 2023 update,” Nucleic Acids Res, vol. 51, no. D1, pp. D1373–D1380, Jan. 2023, https://doi.org/10.1093/nar/gkac956
S. Charleson et al., “Characterization of a 5-lipoxygenase-activating protein binding assay: correlation of affinity for 5-lipoxygenase-activating protein with leukotriene synthesis inhibition.,” Mol Pharmacol, vol. 41, no. 5, p. 873, 1992, [Online]. Available: http://molpharm.aspetjournals.org/content/41/5/873.abstract
M. J. ; T. Frisch G. W.; Schlegel H. B.; Scuseria G. E.; Robb M. A.; Cheeseman J. R.; Scalmani G.; Barone V.; Mennucci B.; Petersson G. A.; Nakatsuji H.; Caricato M.; Li X.; Hratchian H. P.; Izmaylov A. F.; Bloino J.; Zheng G.; Sonnenb Wallingford CT, “Gaussian 09 (Revision A.2).” 2009.
P. Willett, “Similarity Searching Using 2D Structural Fingerprints,” in Chemoinformatics and Computational Chemical Biology, J. Bajorath, Ed., Totowa, NJ: Humana Press, 2011, pp. 133–158. https://doi.org/10.1007/978-1-60761-839-3_5
K. Gao, D. D. Nguyen, V. Sresht, A. M. Mathiowetz, M. Tu, and G.-W. Wei, “Are 2D fingerprints still valuable for drug discovery?,” Physical Chemistry Chemical Physics, vol. 22, no. 16, pp. 8373–8390, 2020, https://doi.org/10.1039/D0CP00305K
J. Duan, S. L. Dixon, J. F. Lowrie, and W. Sherman, “Analysis and comparison of 2D fingerprints: Insights into database screening performance using eight fingerprint methods,” J Mol Graph Model, vol. 29, no. 2, pp. 157–170, 2010, https://doi.org/https://doi.org/10.1016/j.jmgm.2010.05.008
Y. An, W. Sherman, and S. L. Dixon, “Kernel-Based Partial Least Squares: Application to Fingerprint-Based QSAR with Model Visualization,” J Chem Inf Model, vol. 53, no. 9, pp. 2312–2321, Sep. 2013, https://doi.org/10.1021/ci400250c
“Canvas, version 2.1, Schrödinger, LLC, New York, NY,.” 2020.
P. De, S. Kar, P. Ambure, and K. Roy, “Prediction reliability of QSAR models: an overview of various validation tools,” Arch Toxicol, vol. 96, no. 5, pp. 1279–1295, 2022, https://doi.org/10.1007/s00204-022-03252-y
K. Rullah et al., “Identification of Novel 5-Lipoxygenase-Activating Protein (FLAP) Inhibitors by an Integrated Method of Pharmacophore Virtual Screening, Docking, QSAR and ADMET Analyses,” Journal of Computational Biophysics and Chemistry, vol. 22, no. 01, pp. 77–97, 2023, https://doi.org/10.1142/S2737416523500059
H. A. Khan and I. Jabeen, “Combined Machine Learning and GRID-Independent Molecular Descriptor (GRIND) Models to Probe the Activity Profiles of 5-Lipoxygenase Activating Protein Inhibitors,” Front Pharmacol, vol. 13, 2022, https://doi.org/10.3389/fphar.2022.825741
X. Ma, L. Zhou, Z.-L. Zuo, J. Liu, M. Yang, and R.-W. Wang, “Molecular Docking and 3-D QSAR Studies of Substituted 2,2-Bisaryl-Bicycloheptanes as Human 5-Lipoxygenase-Activating Protein (FLAP) Inhibitors,” QSAR Comb Sci, vol. 27, no. 9, pp. 1083–1091, 2008, https://doi.org/https://doi.org/10.1002/qsar.200810053
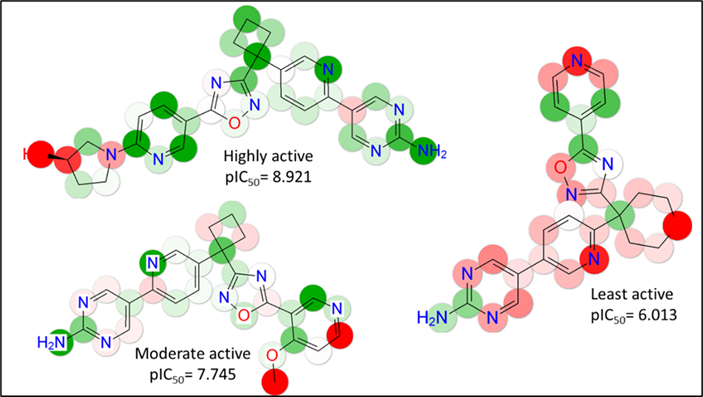
Copyright (c) 2024 Mandeth Kodiyil Geetha Nambiar, Thaikadan Shameera Ahamed (Author)

This work is licensed under a Creative Commons Attribution 4.0 International License.